In this episode, we are joined by Josh Budman, VP of Analytics at Net Health to talk about the future of machine learning in health care and how it will affect provider organizations.
Podcast (hfppodcast): Play in new window | Download
Learn how to listen to The Hospital Finance Podcast® on your mobile device.Highlights of this episode include:
- Differences between artificial intelligence and machine learning
- Current applications of machine learning in health care
- The future of machine learning
- Remote patient monitoring
- Key points in selecting applications or vendors to work with
Mike Passanante: Hi, this is Mike Passanante and welcome back to the award-winning Hospital Finance podcast. On today’s show, I’m joined by Josh Budman, VP of Analytics at Net Health. Josh is a biomedical engineer and founder of Tissue Analytics, which was subsequently acquired by Net Health. On this episode, Josh and I are going to talk about the future of machine learning in health care and how it will affect provider organizations. Josh, welcome to the show.
Josh Budman: Thank you so much, Mike. Pleasure to be here.
Mike: So, Josh, before we talk about the specifics of technology in health care, let’s unpack some terminology. Can you explain for us the differences between artificial intelligence and machine learning?
Josh: Yeah. Absolutely. And it’s a very common question, and I would say that depending on who you ask, there will be slightly different definitions. The best one I’ve heard is that machine learning is a subset of artificial intelligence. So using that statement to set the tone for the rest of what I say, artificial intelligence can blanket any type of intelligence that is executed or logic even that is executed by a machine. So if we’re thinking in the software realm everyone probably is familiar with the term, “if statement”; If this then that. That is a form, if crudely, of artificial intelligence. A robot performing a task that it was programmed to do is also a form of artificial intelligence. But when it comes to machine learning, machine learning is still within the realm of AI but it is basically when you teach a machine or a computer to learn from data. And when I say learn, I specifically mean being able to generally infer and generally make inference from existing data that is out there. And so an example of that is– for instance, let’s try to correlate if someone lives in X location, what would you expect their salary to be? That’s an example of a machine learning problem that could be solved by a simple regression and a pretty common one when it comes to the machine learning space. I know we’ll talk more about machine learning in health care, but that’s how I would say the two differ. They’re related. If you look at the Venn diagram, it would just be a machine learning circle inside the AI circle. That’s how I like to look at it.
Mike: Got it. So, Josh, can you talk about some current applications of machine learning in health care that might already be familiar to us?
Josh: Absolutely. So I think the most familiar to anyone that would be listening to this or anyone out in the wild are those dictation tools that doctors used to actually write down their medical notes from when they speak into a microphone. The most popular of those softwares and probably the one that basically monopolizes that industry is called– the company is called Nuance and the main product is called Dragon. And so that is a very good example of machine learning. To bring it back to my definition, they have a very large data set of medical terminology of past medical notes that they have analyzed and as well as a lot of voice data, and from that data, they’ve been able to basically parse and generalize to create a note from voice. So voice to note. And that inference that’s being performed is a perfect example of a machine learning algorithm in the wild and the health care space. I would say that generally speaking, there is a lag between a lot of the R&D work that is being done in this space and what would be popularly known to the public. There’s a few other software products out there, software companies out there. There’s one called Zebra Medical Vision which does a lot of cool machine learning-based computer vision tools similar to Tissue Analytics, of course, the company that I co-founded and that was acquired like you introduced. And there is also some of the large electronic medical records that I’m sure are discussed several times on the show, like Epic and Cerner, are actually building in machine learning tools into their software. So Epic recently had some publicity around a sepsis risk algorithm that they embedded into their software. And Cerner had a pretty nice publication in press about some digital healthcare tools they were releasing to basically correct errors in transcribing notes from clinicians at Memorial Hermann. So a lot of good stuff coming out. I would say that in the 2020s are when we’ll start to see a lot more of these applications getting into users hands.
Mike: So let’s talk about the future. What do you think is on the horizon in terms of machine learning and health care?
Josh: Another great question. I think that a lot of the algorithms coming out now are predictive in nature where that is, you get an existing set of data and you try to optimize the predictive power of your algorithm. So you get past data and you try to get as accurate as a result on new data that you might receive. I think we’re going to, like I said in my previous answer, start to see a lot more of these applications actually getting into the hands of providers as the electronic medical record software companies open up their doors to integrations, which we started seeing in 2017, 2018. I think that although we’re working on predictive work right now in the R&D space that will get into the hands of providers that the next generation, from an R&D perspective, is prescriptive analytics. So we’re talking instead of just optimizing a result or optimizing a prediction, we’re actually going to be able to show very clearly why that result might take place or did take place. And right now, there’s a lot of mix up between predictive and prescriptive. I think most of what is being released, again if not all, is predictive. Prescriptive will require a higher form of regulatory approval but I do think that is the next frontier of analytics in health care and will be a very good aid to clinicians, even more so even than predictive analytics are.
Mike: Josh, let’s turn to one specific application that’s changing things, and that’s remote patient monitoring. That really has the potential to shift the paradigm of care. What’s your take on that? And how is machine learning applied in that space?
Josh: Yeah. So remote patient monitoring is definitely something that got, as I’m sure you’re very aware, a lot more popular with the pandemic. Early 2020 was when we started to see kind of a very clear boom or very acute boom in these types of applications. I think there is, of course, a ton of value in it. My company, Tissue Analytics, and of course, Net Health, we have remote patient monitoring type applications. What I will say is that this space fails if the actual execution of the software isn’t well integrated with a clinical workflow. So I think a lot of companies of small or even medium to large size were finding that simply being able to enable video conferencing or some two-way messaging system in your application was not really the silver bullet to “pressing the on button” for remote patient monitoring. There has to be some sort of integration with schedule. I think that that’s where a lot of the frantic work to deploy remote patient monitoring and software solutions that hadn’t had it prior to the pandemic failed. And so I do think there is a ton of value in it. I think that we are going to continue to see them improve. And one way that they will improve is, of course, with the augmentation that machine learning will allow. I think that one of those risks, of course, with RPM, a remote patient monitoring, is that you might not be able to get a high enough integrity result from a simple video conference or even teleconference between provider and patient. I think if we have trustworthy machine learning algorithms that are providing data and augmenting the clinical remote experience with that data, it’ll be able to create an infrastructure where remote patient monitoring plus that machine learning algorithms result is actually enough to match the quality of care that a provider with human touch would be able to provide. You’re seeing that in certain areas, but I think that’s the element that machine learning combined with remote patient monitoring would provide.
Mike: Okay. Josh, as providers select applications or vendors to work with, how can they evaluate if machine learning or other technologies are actually bringing value to the table? It seems like everyone’s claiming to use AI or RPA or something else to fuel their solutions. What are your thoughts on that?
Josh: Yeah. Of course, we all know that these are buzzwords that analytics, machine learning, big data, etc., are buzzwords that are said a lot, I probably am being repetitive by even calling them buzzwords. I’m sure that several guests previously have even said that. I think that’s fundamentally, especially in health care, where these tools succeed or fail is in how well they’re integrated with the clinical workflows. Similar to RPM, the quality of an algorithm only goes so far as its ability to be integrated with existing clinical workflows. Providers are extremely busy. They’re tasked with making sure their outcomes are optimal and so giving them a very accurate algorithm that they have to take a ton of extra steps or even a few extra steps to access is effectively worthless. And so I think when health care systems or other groups are evaluating quality of a technology in addition to its accuracy and in addition to the data set that powers those algorithms, I think they have to actually evaluate how well integrated that technology will be with their existing workflows and whether or not that technology actually allows them to have improved or at least not diminished workflows. And that’s, I’d say, something that I’ve learned over the years with Tissue Analytics and now even more so with Net Health. It’s so crucial to make sure that your machine learning algorithm doesn’t compromise the integrity of your workflows.
Mike: That’s great advice. Josh, if someone wanted to learn more about you or the work you do, where can they go?
Josh: Yeah. So you can find me personally on LinkedIn, Joshua Budman. Our parent company, Net Health is nethealth.com. We are about to go general availability, GA, with some of our first predictive algorithms. Again, to back to my last answer, directly embedded within our electronic records software so we don’t compromise workflow at all with those. You’ll start to see those being advertised publicly as well as on the website over time. And then if you want to find out more about the company that I started and was acquired and is still operating as a wholly-owned subsidiary, that would be tissue-analytics.com. So any of those sites and I hope that you find that content interesting.
Mike: Exciting times, indeed. Josh Budman, thanks for joining us today on the Hospital Finance podcast.
Josh: Thank you, Mike. Pleasure to talk to you.
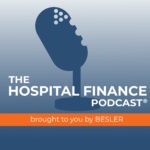